I’ve just downloaded a lot of points — all peaks of the Alps — from Openstreetmap using the QuickOSM plugin in QGIS (search key: natural, value: peak). But to avoid connection timeouts, I had to narrow down my area of interest for each request. Now I want to combine the resulting QGIS layers (e.g. as shapefiles) into one layer, without the duplicates. This would be tricky in QGIS, but Python comes to help.
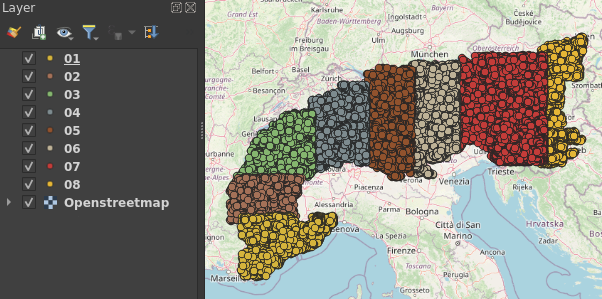
The following code is available as Jupyter notebook on GitHub.
The data used in this notebook is © OpenStreetMap under the Open Database License.
In a second post, I explain how to check if a feature is within a polygon (e.g. within a country or region).
Load all shapefiles into one GeoDataFrame and remove duplicates
import pandas as pd import geopandas as gpd import os folder = 'alpinepeaks/' # Create a list of all shapefiles # (Change the code to use other formats such as geopackage) filelist = [] for file in os.listdir(folder): if file.endswith(('.shp')): filelist.append(file) # Read all shapefiles into a single geopandas GeoDataFrame gdf = gpd.read_file(folder + filelist[0]) gdf.set_index('full_id', inplace=True) for file in filelist[1:]: newgdf = gpd.read_file(folder + file) newgdf.set_index('full_id', inplace=True) gdf = pd.concat([gdf, newgdf]) # remove duplicates gdf = gdf[~gdf.index.duplicated(keep='first')]
Clean data
Chances are, we have a lot of useless columns populated mostly by “None”. Let’s only keep data we really care about. This is best done in a jupyter notebook or the python shell, in order to distinguish the data to be dropped.
# First, I remove rows without name (I don't need them) gdf = gdf[gdf['name'].notna()] # How many columns do we have? len(list(gdf.columns)) # List all columns (sorted) sorted(list(gdf.columns)) # To check for e.g. 'outdoor': gdf[gdf['outdoor'].notna()].dropna(axis=1) # Only two rows # Only keep useful columns useful = ['name', 'prominence', 'ele', 'geometry', 'name_en', 'name_fr', 'name_it','alias', 'alt_name', 'alt_name2', 'sport', 'name_1', 'name_de_AT', 'name_de_DE'] gdf = gdf[useful]
Save
I save the result as geojson:
gdf.to_file(folder + "peaks.geojson", driver='GeoJSON')
Read on
How to check if a map feature is within a polygon (e.g. within a country) using Python